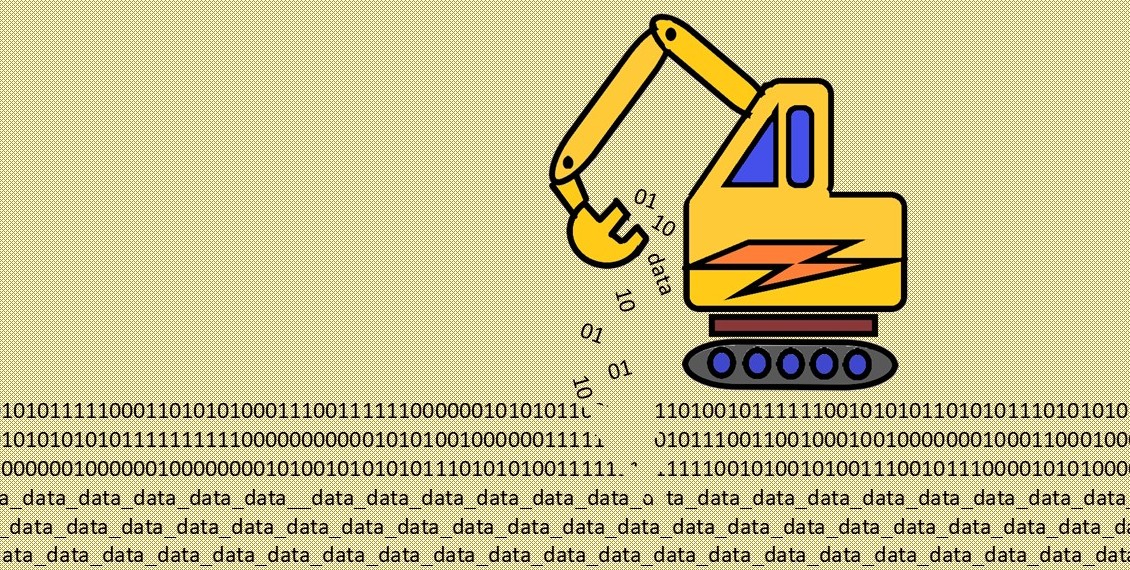
Once an element of science fiction, machine learning (ML) and artificial intelligence (AI) have now become intrinsic to our daily lives. Aiding and abetting that proclivity, comes the enormous amount of data that is generated every day, waiting to be analyzed and put to use by ML techniques. In fact, several studies suggest that more than 90% of the data available in the world today was generated in the last two years alone. However, machine learning is not limited to suggesting map routes, recognizing voices and other such everyday engineering feats. Basic sciences and research too have been deeply impacted. Scientific research these days can be guided not just by hypothesis-based, but also by data-driven approaches. Now that BPS 2019 meeting has drawn to a close, it is time to analyze, have we biophysicists caught up?
A quick (and regretfully crude) look seems to suggest a promising trend. Searching by the terms ‘machine learning’, ‘artificial intelligence’, ‘deep learning’ and ‘big data’ in the abstract issues of BPS meetings of the last three years show the following number of hits of independent abstracts or exhibitor presentations: 4 in 2016, 6 in 2017, and 9 in 2018. Yet, the same search gives 45 positive and independent hits in the 2019 program! Moreover, several other presentations had some degree of ML being utilized, even though it was not mentioned in the abstract or title. Times are changing, and how! Even the organizers have shifted from pdf and printed versions of the program to a mobile app for the conference planner.
This BPS meeting saw intense ML applications in a variety of fields: genetic mutational and sequence based studies, feature detection and dimensional reductions in conformational spaces, studying complex kinetics, force-field parameterization for simulations, and the list continues. Even experimental techniques have tied up with deep learning methods to aid in analyses. Different microscopy techniques, pharmacology studies, bio-nanosensor design – all are now benefitting by combining complex experimental studies with data-driven analytical approaches. Furthermore, two out of the five workshops at the meeting were Big-Data oriented: “The role of data resources in biophysics” and “Squeezing the most out of your data – Bayesian statistical inference for biophysics”. Even being scheduled late on Tuesday evening, these workshops saw packed audiences. With the Moore’s law-like growth in data, it can be assumed that this trend is here to stay for BPS.
These are revolutionary times in the field of biophysics. We as scientists just need to make sure that there is extensive collaboration and data sharing. More importantly, let us strive towards careful vetting of data collection conditions and compatibilities to make the most of these techniques, rather than hastily jumping on to the bandwagon simply for the sake of it.